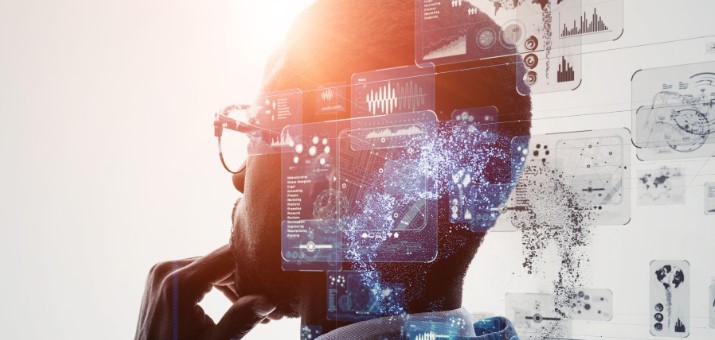
For decades, marketers have grappled with the age-old question: “Which marketing channel is driving sales?” While traditional market mix modeling (MMM) techniques have offered statistical correlations, they often fall short in pinpointing the causal impact of each marketing effort. Enter AI-powered causal learning using neural networks – a disruptive force reshaping the MMM landscape in 2024 and beyond.
The Limitations of Traditional MMM:
Traditional MMM approaches, relying on linear regression or econometric models, attempt to quantify the impact of each channel based on historical data. However, these models struggle with:
- Multicollinearity.
- Granularity of the reads.
- Interactions between different tactics.
- Limited predictive power: Traditional MMM often struggles to accurately predict future outcomes, hindering proactive campaign optimization.
- Confounding variables: External factors like seasonality or economic trends can skew results, making it difficult to isolate the true impact of marketing efforts.
Enter the AI Causal Revolution:
AI causal learning using neural networks tackles these limitations head-on. These algorithms, inspired by the human brain, can sift through mountains of data, identifying hidden patterns and causal relationships between marketing activities and sales outcomes. They go beyond correlation, offering insights into the “why” behind customer behavior, not just the “what.”
Here’s how AI neural networks redefine MMM:
- Addresses Collinearity and dimensionality by learning nonlinearity and interactions.
- Unmasking confounding variables: AI can isolate the true impact of marketing efforts by statistically accounting for external factors that might influence sales.
- Advanced attribution models: Neural networks can untangle the web of customer interactions, attributing sales credit across multiple touchpoints based on their causal influence.
- Precise predictions: By learning from complex data patterns, AI models can predict future sales with greater accuracy, enabling smarter campaign budgeting and optimization.
Real-world applications in 2024:
The implications for marketers are staggering. Imagine:
- Optimizing campaign spend: AI can pinpoint underperforming channels and reallocate budget to proven drivers of sales, maximizing ROI.
- Personalizing marketing efforts: By understanding audience level ROI, campaigns and promotions can be planned for higher engagement and conversion rates.
- Identifying hidden growth opportunities: AI can uncover previously unsuspected relationships between marketing activities and sales, paving the way for innovative, data-driven strategies.
The Human in the Loop:
It’s crucial to remember that AI is not a magic wand. While it provides powerful insights, human expertise remains essential for interpreting results, making strategic decisions, and ensuring ethical marketing practices. The future of MMM lies in a harmonious collaboration between human intuition and AI-powered causal learning.
Conclusion:
In 2024, AI causal learning using neural networks is poised to fundamentally transform market mix modeling. By untangling the causal web of customer behavior, it offers unparalleled insights and predictive power, paving the way for a new era of data-driven marketing excellence. However, navigating this exciting frontier requires embracing ethical considerations, prioritizing human oversight, and recognizing AI as a powerful tool, not a replacement for human ingenuity. Are you ready to unlock the causal secrets of your marketing success? The AI revolution is here, and it’s time to join the dance.
To know more about us write to info@datapoem.com